The term ‘AGI’ has been badly distorted and abused, be it out of ignorance or for fund-raising purposes. It properly refers to systems that can autonomously learn to perform novel human-level cognitive tasks given limited resources of time and compute; essentially what a smart college graduate could learn by ‘hitting the books’. They could learn to become a skilled programmer, accountant, or research scientist.
From this it follows that:
a) AGI will be even more valuable than electricity
b) Deep Learning and Large Language Models (LLMs) won’t get us there
So why, in spite of tens of billions of dollars and thousands of Ph.D.-level AI researchers working on this for 10 years or more, don’t we have a clear, obvious, and generally acceptable path to AGI yet?
A one-sentence summary is that the tremendous success of Big Data approaches over the past 10 years has ‘sucked all of the oxygen out of the air’, and viable alternatives have not been pursued by enough people with meaningful resources.
A more detailed analysis exposes several common key mistakes that have prevented meaningful progress towards Real AGI. I call them ‘The 7 Deadly Sins of AGI design’.
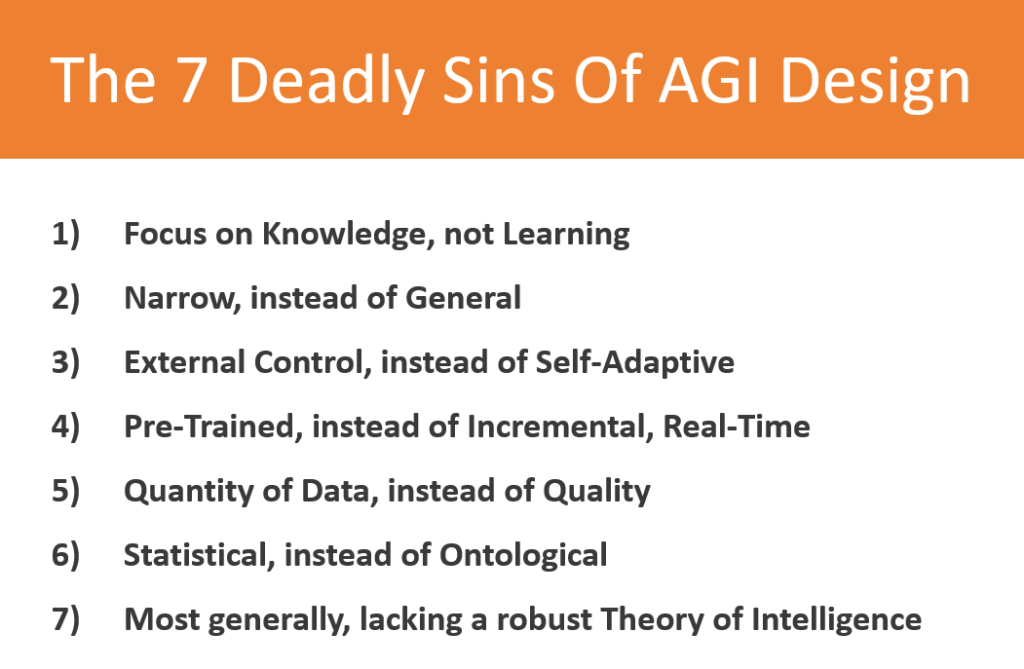
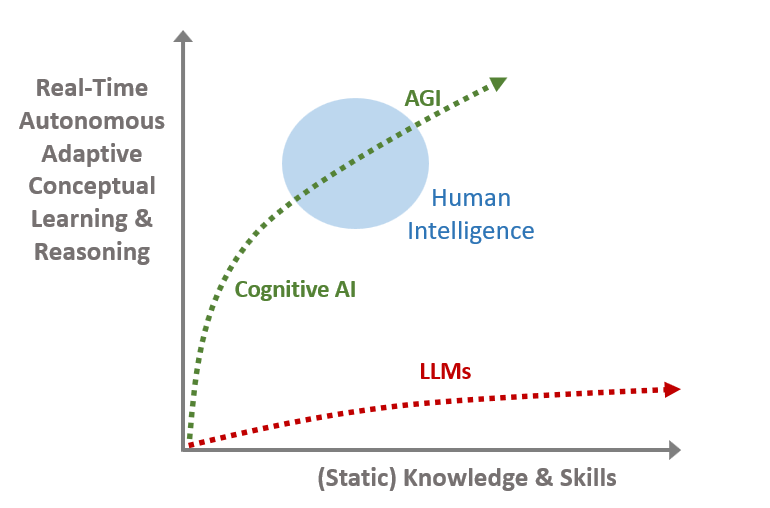
Once training is complete and the model is put into production the core model cannot be updated incrementally in real time — it is read-only! This means that any changes in the real world (like elections, conflicts, scientific breakthroughs, etc.) since the beginning of training cannot be part of the model. This is a very serious limitation. One can hardly consider a system to be intelligent if it cannot adjust its core knowledge given changing circumstances.
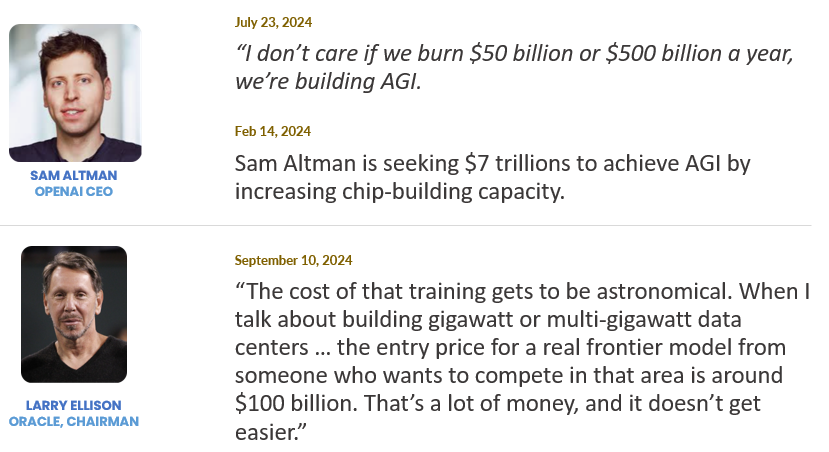
A much more reasonable approach would be to first deeply explore and understand the essentials of intelligence and how the human mind works – how we learn and reason. Such research would cover epistemology (theory of knowledge), the nature of knowledge and how we obtain certainty. More broadly, a philosophical understanding of consciousness, volition, and ethics (how we know wrong from right). It would include a thorough grasp of cognitive psychology: How our intelligence differs from other animals’. How children learn most effectively. What (good) IQ tests measure.
Without a good theory of human intelligence AGI will at best be stumbled across by accident. By their own admission, none of the current AI leaders have a theory or plan on how to achieve AGI.
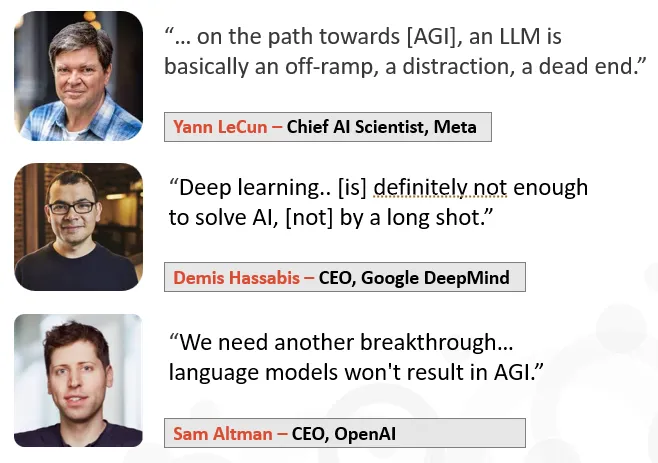
The Seven Sins — Almost all current AI projects commit all or most of these sins. We will not achieve AGI without taking them seriously. One very different approach is what DARPA calls the ‘Third Wave of AI’, or Cognitive AI. It is a much smarter path to AGI. It requires orders of magnitude less data and compute, and has the ability to learn incrementally in real-time. An example of this is Aigo.ai’s Integrated Neuro-Symbolic Architecture.
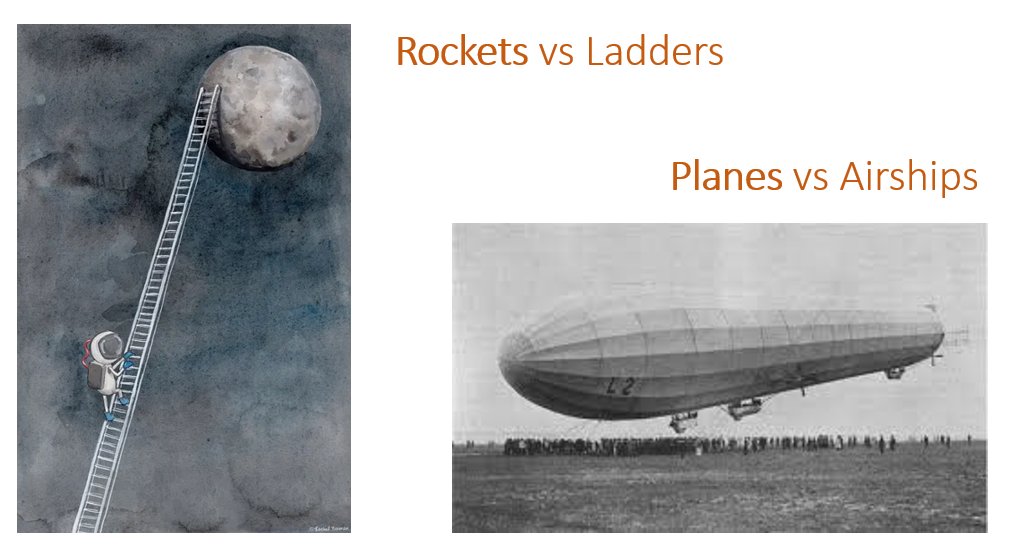